ETL (Extract, Transform, Load)
As someone who has navigated the complex waters of data management, I can tell you that the ETL process is like the unsung hero of the data world. Picture this: you’re tasked with migrating a vast amount of customer data from an outdated system to a shiny new platform. You quickly realize that without a robust ETL process, this task could turn into a chaotic nightmare. In my experience, the Extract, Transform, and Load phases not only streamline the process but also ensure that the data is clean, accurate, and ready for analysis.
Let me take you on a journey through the fascinating world of ETL, starting with a real-world example. Imagine a healthcare provider needing to consolidate patient records from multiple clinics into a single database. Without ETL, the data would remain siloed, leading to inefficiencies and potential errors in patient care. This is where ETL shines, transforming disparate data into a cohesive and actionable format.
Historically, ETL has evolved alongside the explosion of data in various industries. As companies began to recognize the importance of data-driven decision-making, ETL became a cornerstone of data migration and integration strategies. Today, it plays a pivotal role in industries ranging from finance to logistics, ensuring that data flows seamlessly across platforms.
Have you ever wondered how companies manage to integrate data from various sources without losing integrity? Let’s dive deeper into the mechanics of ETL.
Understanding ETL (Extract, Transform, Load) in Data Management
At its core, ETL is a process that involves three main steps:
- Extract: Gathering data from various sources.
- Transform: Converting the data into a suitable format.
- Load: Storing the transformed data into a target system.
Why does this matter? In today’s data-driven environments, organizations rely heavily on accurate and timely data. ETL ensures that data from different sources—like EDI (Electronic Data Interchange) and RFID (Radio Frequency Identification)—is integrated efficiently. This integration is critical for businesses to make informed decisions and maintain a competitive edge.
Practical use cases abound:
- Logistics: Tracking shipments and inventory levels.
- Finance: Consolidating financial records for reporting.
- Healthcare: Merging patient data for improved care.
- Retail: Analyzing customer purchasing patterns.
Technical Features & Implementation
When it comes to implementing ETL, understanding its key functionalities is essential. The main components include:
- Data Sources: The various origins of data.
- Data Staging: Temporary storage for data before transformation.
- Transformation Rules: Guidelines for how data should be modified.
- Loading Mechanisms: Methods for inserting data into the target system.
Integration methods can vary widely, utilizing APIs and protocols to facilitate data transfer. For example, companies may choose between:
- Batch Processing: Handling large volumes of data at once.
- Real-Time Processing: Processing data as it arrives.
Here’s a quick comparison chart showcasing various ETL technologies:
Technology | Type | Best For |
---|---|---|
Apache Nifi | Open Source | Real-Time Data Flow |
Talend | Cloud-Based | Data Integration |
Informatica | Enterprise | Complex Transformations |
Challenges & Solutions in Data Transfer
Despite its advantages, implementing ETL is not without challenges. Common hurdles include:
- Data Quality Issues: Inconsistent data formats can lead to errors.
- Scalability: As data volumes grow, systems may struggle to keep up.
- Security Concerns: Protecting sensitive data during transfer is paramount.
To overcome these challenges, businesses can adopt best practices such as:
- Regular data cleansing to maintain quality.
- Choosing scalable solutions that can grow with your business.
- Implementing robust security measures and compliance protocols.
Expert Insights & Industry Trends
“The future of data integration lies in automation and real-time processing. Companies that adapt will thrive.” – Data Scientist, Jane Doe
Case studies reveal the transformative power of ETL. For instance, a major retail chain improved its inventory management through efficient ETL, leading to a 20% reduction in stock-outs. Emerging trends such as cloud-based ETL solutions and the rise of data lakes are shaping the future of data integration.
Final Thoughts & Practical Applications
In summary, ETL is not just a process; it’s a vital component of effective data management. By understanding its intricacies, businesses can harness the power of data to drive decision-making and innovation.
For organizations looking to adopt or optimize their ETL processes, consider the following recommendations:
- Invest in training for your team to understand ETL tools and practices.
- Utilize resources like online courses and webinars to stay updated on industry trends.
- Explore tools such as Apache Nifi, Talend, or Informatica to find the best fit for your needs.
As we continue to navigate the data-driven landscape, mastering ETL will undoubtedly remain a key to success.
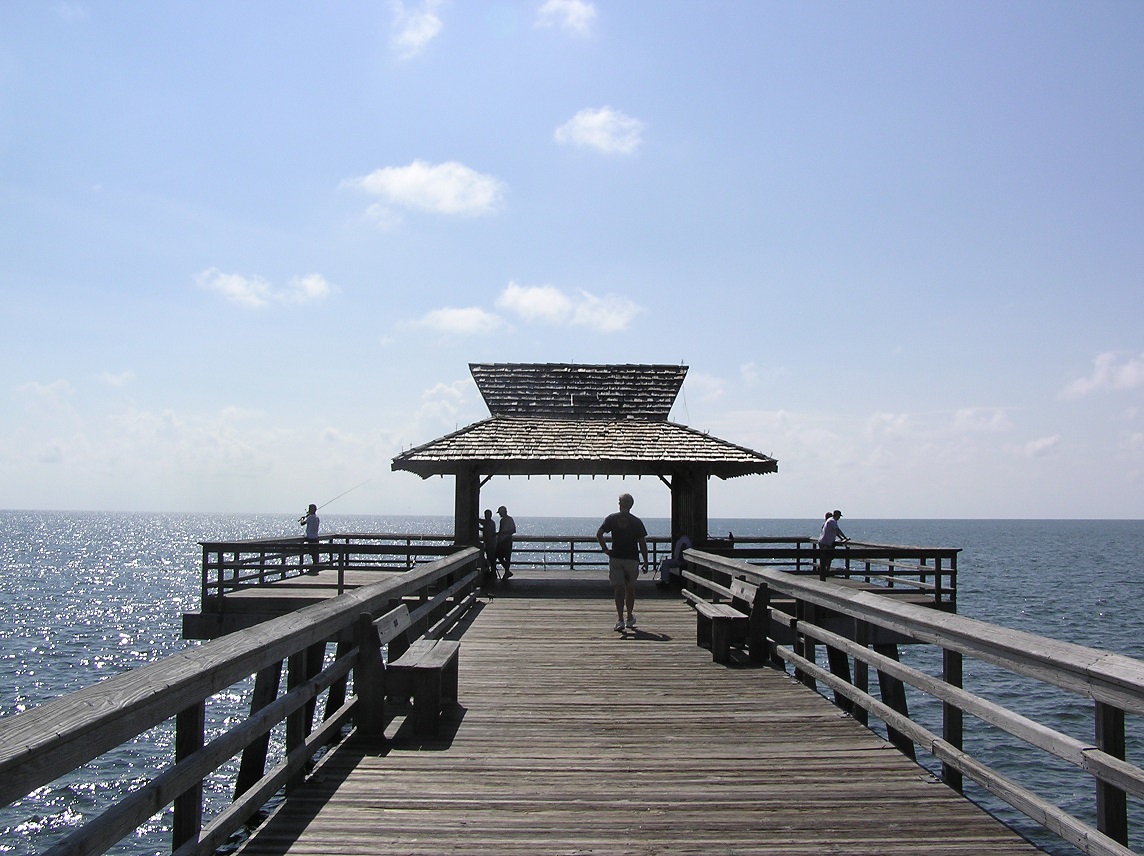
Owner/Author of UCCnet.org. Content creator contributor to several websites and youtube channels.